1. Introduction:The Advent of Claude 4 and the New Era of Autonomous AI
The artificial intelligence landscape is one of perpetual,rapid evolution,where each breakthrough redefines the boundaries of possibility. On **May 22,2025**,Anthropic,a research company at the forefront of AI safety and capability,unveiled its latest and most potent creation:the **Claude 4 model family**. This family includes the flagship **Claude Opus 4**,engineered for peak performance in the most demanding tasks,and the highly efficient **Claude Sonnet 4**,designed for scaled deployment and high-throughput applications. This launch is not merely an incremental step;it heralds a significant generational leap,aiming to establish new state-of-the-art (SOTA) benchmarks in critical domains such as software engineering,complex logical reasoning,and,most notably,the development and operation of sophisticated autonomous AI agents.
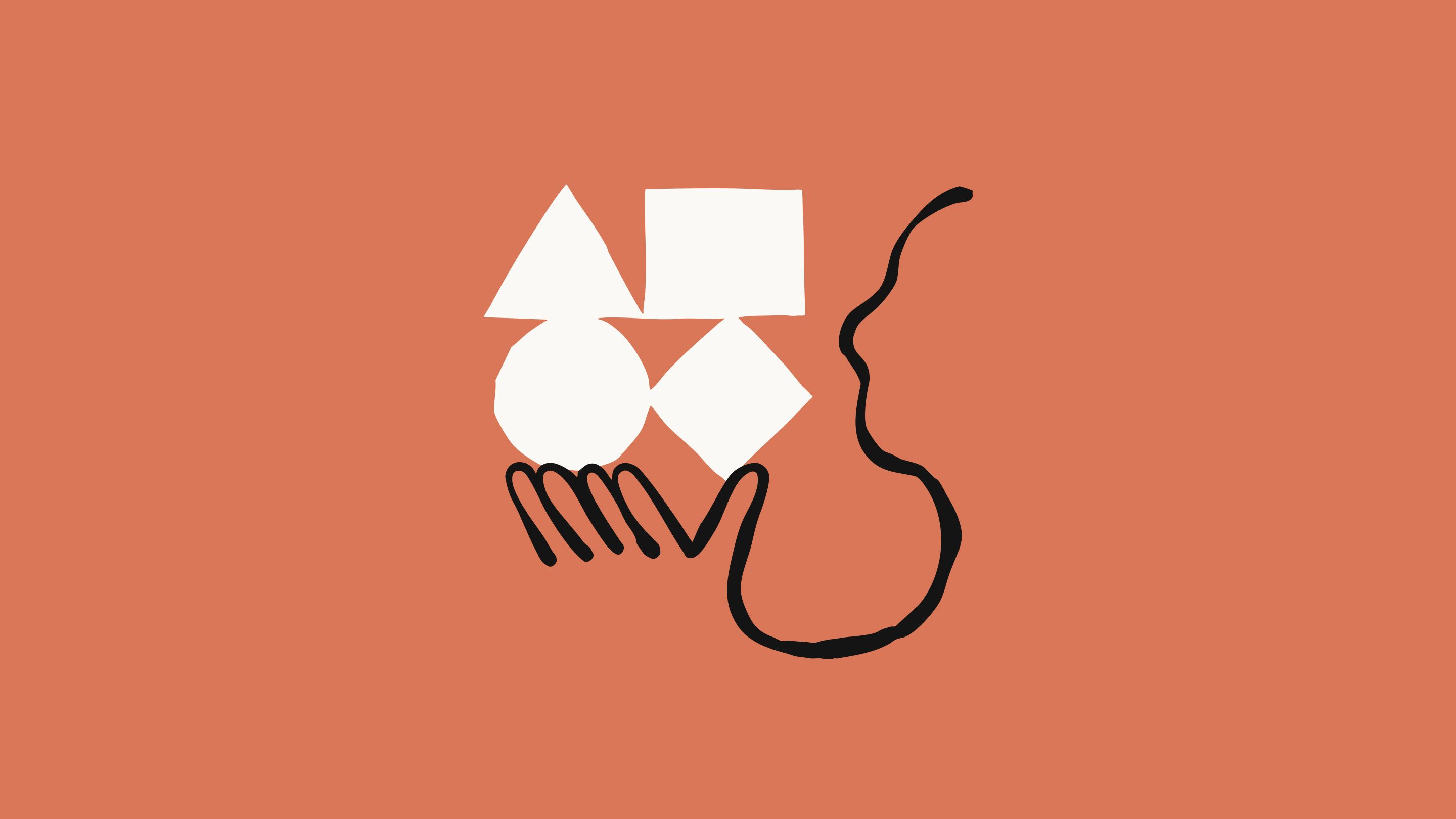
Anthropic positions Claude 4 as its most consequential release to date,meticulously crafted to address the escalating demands of enterprise users,developers,and researchers who require AI systems capable of more than just pattern recognition or text generation. The core promise of Claude 4 lies in its ability to engage in **extended,coherent reasoning**,to understand and manipulate vast quantities of information (courtesy of its **200K+ token context window**),to utilize external tools with unprecedented **parallelism and efficiency**,and to operate autonomously for extended periods—reportedly up to **seven hours on complex coding tasks** for Opus 4.
The strategic timing and simultaneous launch across premier cloud platforms,including **Amazon Bedrock** and **Google Cloud Vertex AI**,underscore Anthropic's commitment to making these powerful tools immediately accessible and integrable into existing enterprise workflows. This move aims to lower adoption barriers and empower organizations to leverage Claude 4's capabilities for tangible productivity gains,innovation acceleration,and the creation of novel AI-driven solutions.
This comprehensive analysis will embark on a deep dive into every facet of Claude 4. We will dissect its official launch details and strategic rationale,meticulously examine its revolutionary features and the technical innovations that power them,and scrutinize its performance across a battery of industry-standard benchmarks. Furthermore,we will place Claude 4 within the competitive landscape,comparing its strengths and weaknesses against other leading AI models. The article will also explore the transformative impact Claude 4 is poised to have across various industries,delve into Anthropic's approach to AI safety and ethics with such powerful models,and peer into the future roadmap for the Claude AI series. Our goal is to provide a definitive resource for understanding not just *what* Claude 4 is,but *why* it matters and *how* it is set to reshape the future of artificial intelligence and its application in the real world.
For the Tech-Savvy Reader: Throughout this article,we will endeavor to provide as much technical depth as publicly available information and reasonable inference allow,discussing potential architectural underpinnings,computational considerations,and the nuances of AI model evaluation. We aim to satisfy the curiosity of developers,researchers,and AI practitioners seeking a thorough understanding of Claude 4's capabilities.
2. Official Launch Details & Strategic Imperatives
The arrival of Claude 4 on May 22,2025,was a meticulously orchestrated event,signaling Anthropic's ambitious plans to redefine the SOTA in AI and capture significant mindshare among developers and enterprise clients. The launch strategy,encompassing model variants,platform availability,and initial positioning,reveals a deep understanding of the current AI market dynamics and user needs.
The Dual Model Strategy:Opus 4 and Sonnet 4
Anthropic introduced two distinct models under the Claude 4 banner,catering to different performance requirements and cost considerations:
Model Variant | Launch Date | Primary Positioning & Target Use Cases | Key Strengths & Differentiators | Initial Availability Platforms |
---|---|---|---|---|
Claude Opus 4 | May 22,2025 | Flagship model delivering peak performance for the most complex AI tasks. Targeted at research,advanced software development,strategic analysis,and sophisticated agentic workflows requiring maximum intelligence. |
| Anthropic API,Amazon Bedrock,Google Cloud Vertex AI. |
Claude Sonnet 4 | May 22,2025 | High-performance,cost-effective model balancing intelligence with speed and efficiency. Ideal for large-scale enterprise deployments,data processing,high-volume coding tasks,and intelligent automation. |
| Anthropic API,Amazon Bedrock,Google Cloud Vertex AI. |
This dual-model approach allows Anthropic to address a broader spectrum of the market. Opus 4 serves as the technology spearhead,demonstrating the pinnacle of AI capability,while Sonnet 4 provides a pragmatic,scalable option for widespread adoption,making advanced AI more accessible for diverse enterprise applications.
Strategic Rationale:Enterprise-Grade Accessibility and Multi-Cloud Deployment
The decision to launch Claude 4 simultaneously on leading cloud platforms like **Amazon Bedrock** and **Google Cloud Vertex AI**,in addition to its own API,is a cornerstone of Anthropic's enterprise strategy. This multi-cloud approach offers several profound advantages:
- Reduced Friction for Adoption: Enterprises often have established relationships and infrastructure with specific cloud providers. Offering Claude 4 natively within these environments significantly simplifies procurement,integration,and management,bypassing the need for organizations to build custom integrations or manage separate vendor relationships for foundational AI models.
- Data Gravity and Proximity: Many organizations store vast amounts of data in the cloud. Running AI models on the same platform where data resides minimizes latency,reduces data transfer costs,and can enhance security by keeping data within an existing compliant environment. This is crucial for tasks involving large datasets,such_as training custom models or processing extensive document repositories with Claude 4's 200K context window.
- Scalability and Reliability: Leveraging the robust,auto-scaling infrastructure of AWS and Google Cloud ensures that enterprises can deploy Claude 4-powered applications with high availability and performance,dynamically scaling resources based on demand. This is critical for production workloads.
- Security and Compliance: Cloud providers offer a suite of security tools,identity management services (like IAM),and compliance certifications. By integrating Claude 4 into these platforms,Anthropic allows enterprises to apply their existing security policies and governance frameworks to AI model usage,addressing critical concerns for regulated industries.
- Access to Complementary Services: Cloud platforms provide a rich ecosystem of other services (databases,MLOps tools,analytics services,serverless functions) that can be easily combined with Claude 4 to build sophisticated end-to-end AI solutions. For example,an application might use Claude 4 on Bedrock to analyze text,store results in Amazon S3,and trigger workflows using AWS Lambda.
- Market Reach and Competition: This strategy directly positions Anthropic to compete with other major model providers like OpenAI (often accessed via Azure) and Google's own Gemini models on Vertex AI. By being present on multiple major clouds,Anthropic maximizes its market visibility and provides customers with choice and flexibility,preventing vendor lock-in to a single AI provider's ecosystem.
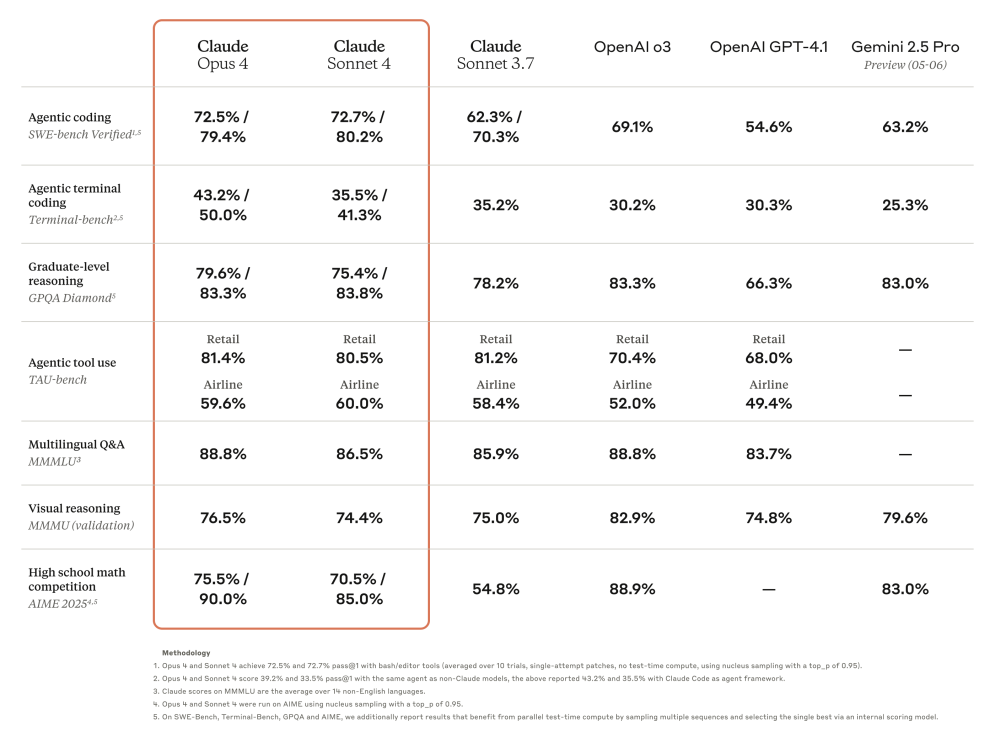
In essence,Anthropic's launch strategy for Claude 4 is not just about showcasing a powerful new AI model;it's about delivering an enterprise-ready solution that is easily accessible,deployable,and manageable within the environments where businesses already operate. This pragmatic approach is designed to accelerate the adoption of Claude 4 for transformative use cases across software development,research,business process automation,and beyond,solidifying Anthropic's position as a key player in the rapidly evolving AI landscape.
Market Traction & Financial Momentum
The launch of Claude 4 builds upon significant market momentum for Anthropic. Reports leading up to the release indicated strong financial performance,with the company's annualized revenue reportedly reaching **$2 billion in Q1 2025**. This represents a substantial increase,potentially doubling from previous periods,signaling robust demand for Anthropic's models and a successful monetization strategy even before the introduction of its most powerful offerings to date.
Anthropic Annualized Revenue Growth (Illustrative)
This financial strength provides Anthropic with the resources to continue its ambitious research and development agenda,further refine its models,expand its infrastructure,and invest in safety research – all critical elements for sustaining leadership in the competitive AI field. The strong revenue figures also serve as a testament to the perceived value and utility of its existing models,setting high expectations for the adoption and impact of Claude 4.
3. Core Features & Innovations:Powering the Next Generation of AI
Claude 4 is not merely an incremental update;it's packed with a suite of groundbreaking features and significant enhancements designed to enable more sophisticated,reliable,and genuinely autonomous AI capabilities. These advancements stem from Anthropic's deep research into model architecture,training methodologies,and AI safety. Let's explore the most impactful innovations that define Claude Opus 4 and Claude Sonnet 4.
3.1 Hybrid Reasoning Modes:Balancing Speed with Depth
A cornerstone of Claude 4's enhanced cognitive abilities is its sophisticated **Hybrid Reasoning system**. This system allows the models to dynamically switch between two primary modes of operation:
- "Instant" Mode (Near-Instant Interactive Responses): For queries that are straightforward or require rapid information retrieval,Claude 4 can respond with remarkable speed,making it ideal for interactive applications like chatbots,quick code suggestions,or real-time data lookup. This mode likely relies on highly optimized pathways within the model that prioritize low latency.
- "Extended Thinking" Mode: When faced with complex problems,ambiguous prompts,or tasks requiring multi-step analysis,planning,and tool integration,Claude 4 can engage its "Extended Thinking" capabilities. This mode allows the model to perform more thorough,deliberative processing,akin to a human spending more time to reason through a challenging issue.
Technical Underpinnings and Operation:
While Anthropic hasn't detailed the precise architecture,"Extended Thinking" likely involves several advanced mechanisms:
- Iterative Refinement: The model might internally generate and critique multiple lines of reasoning or potential solutions before settling on a final output. This could involve internal "scratchpad" mechanisms or chain-of-thought processes that are more elaborate than in previous models.
- Strategic Tool Use Integration: "Extended Thinking" is explicitly designed to incorporate tool use. When the model determines that external information or computation is needed (e.g.,via web search,code execution,or API calls),it can pause its internal generation,invoke the necessary tool(s),process the results,and then resume its reasoning with the new information. This is crucial for grounding responses in factual data or executing complex actions.
- Resource Allocation: This mode might consume more computational resources and take longer to produce a response,representing a trade-off for higher quality and more comprehensive answers to difficult questions. The model likely has an internal mechanism to decide when to invoke this more resource-intensive mode based on the complexity of the input or task.
Practical Examples & Benefits:
- Complex Coding Challenge: Asked to design a novel algorithm for a specific problem,Claude 4 might use "Extended Thinking" to break down the problem,research existing approaches (if tool use is enabled),draft pseudo-code,refine it,consider edge cases,and then generate the final code. An "Instant" mode might offer a quicker,more common solution that might not be as optimal.
- Strategic Business Query: "Analyze the potential impact of X new regulation on our Y market segment and propose three mitigation strategies." This would almost certainly trigger "Extended Thinking," involving understanding the regulation,assessing its implications (possibly by searching for analyses),and then formulating strategic responses.
- Scientific Research Assistance: "Summarize recent advancements in quantum computing related to drug discovery and identify three promising,under-explored research avenues." This demands deep comprehension,synthesis of multiple sources,and creative insight – all hallmarks of "Extended Thinking."
The Hybrid Reasoning system allows Claude 4 to be both a nimble assistant for quick tasks and a profound thinker for complex challenges,optimizing performance and resource utilization dynamically. This adaptability is a significant step towards more human-like cognitive flexibility in AI.
3.2 Advanced Parallel Tool Execution:Supercharging Agentic Workflows
One of the most transformative advancements in Claude 4 is its ability to perform **parallel tool use**. This means the model can invoke and manage multiple tools or external API calls *simultaneously*,rather than being restricted to sequential,one-at-a-time execution. This capability drastically accelerates complex workflows and unlocks new possibilities for AI agents.
How Parallel Tool Use Works (Conceptual):
Imagine a complex user request that requires information from multiple sources and several computational steps. With parallel tool use,Claude 4 can:
- Decompose the Task: Identify sub-tasks that can be performed independently. For example,"Compare the Q1 financial performance of Company A,B,and C,and also get the latest stock price for each." This involves three separate financial data lookups and three stock price lookups.
- Initiate Parallel Calls: Instead of querying for Company A's financials,then Company B's,then C's,and then repeating for stock prices,Claude 4 can initiate calls to the relevant financial data APIs and stock price APIs for all three companies concurrently.
- Manage Asynchronous Responses: The model needs a sophisticated internal mechanism to manage these asynchronous operations,track which tool calls are pending,and handle their responses as they arrive (which may be out of order).
- Synthesize Results: Once all necessary data is retrieved,Claude 4 synthesizes the information from the parallel tool executions to formulate a comprehensive answer.
Conceptual Diagram:Parallel Tool Execution with Memory Integration
Supported Tools:
The synthesis document mentions specific tools Claude 4 can leverage,including:
- Code Execution Tool: For running and testing code snippets,essential for development and debugging tasks.
- MCP Connector (Multi-Capability Platform Connector): This likely refers to a generic framework for integrating with various enterprise APIs and data sources,enabling Claude 4 to interact with diverse internal systems.
- Files API: Allows Claude 4 to read from and write to specified files,crucial for its "Enhanced Memory" feature (discussed next) and for processing user-provided documents.
- Web Search: (Implicitly through "extended thinking") For accessing up-to-date information from the internet.
Benefits and Use Cases:
- Drastic Speed Improvements: For tasks requiring multiple external lookups or operations,parallel execution can cut down completion time significantly compared to sequential processing.
- More Complex Agentic Behavior: Agents can perform more sophisticated actions by orchestrating multiple tools at once. For example,an agent planning a trip could simultaneously check flight availability,hotel prices,and weather forecasts for multiple destinations.
- Enhanced Data Aggregation and Analysis: An agent could query multiple databases,APIs,and web sources in parallel to gather comprehensive data for a report or analysis.
- Real-time Decision Making: In dynamic environments,the ability to quickly gather information from several sensors or sources in parallel can enable faster and more informed decisions.
Parallel tool use is a significant architectural achievement,moving AI agents closer to the efficiency of human multitasking in information gathering and action execution. It's a key enabler for the advanced autonomous capabilities highlighted in Claude 4.
3.3 Enhanced Memory with File Capability:Achieving Long-Term Context and Persistence
While large context windows (like Claude 4's 200K tokens) are excellent for handling information within a single,continuous interaction,true long-term memory and persistence across sessions or very long tasks require more. Claude 4 introduces an **Enhanced Memory system,notably through its ability to create and access "memory files"** when granted permission to interact with a local file system (via the Files API).
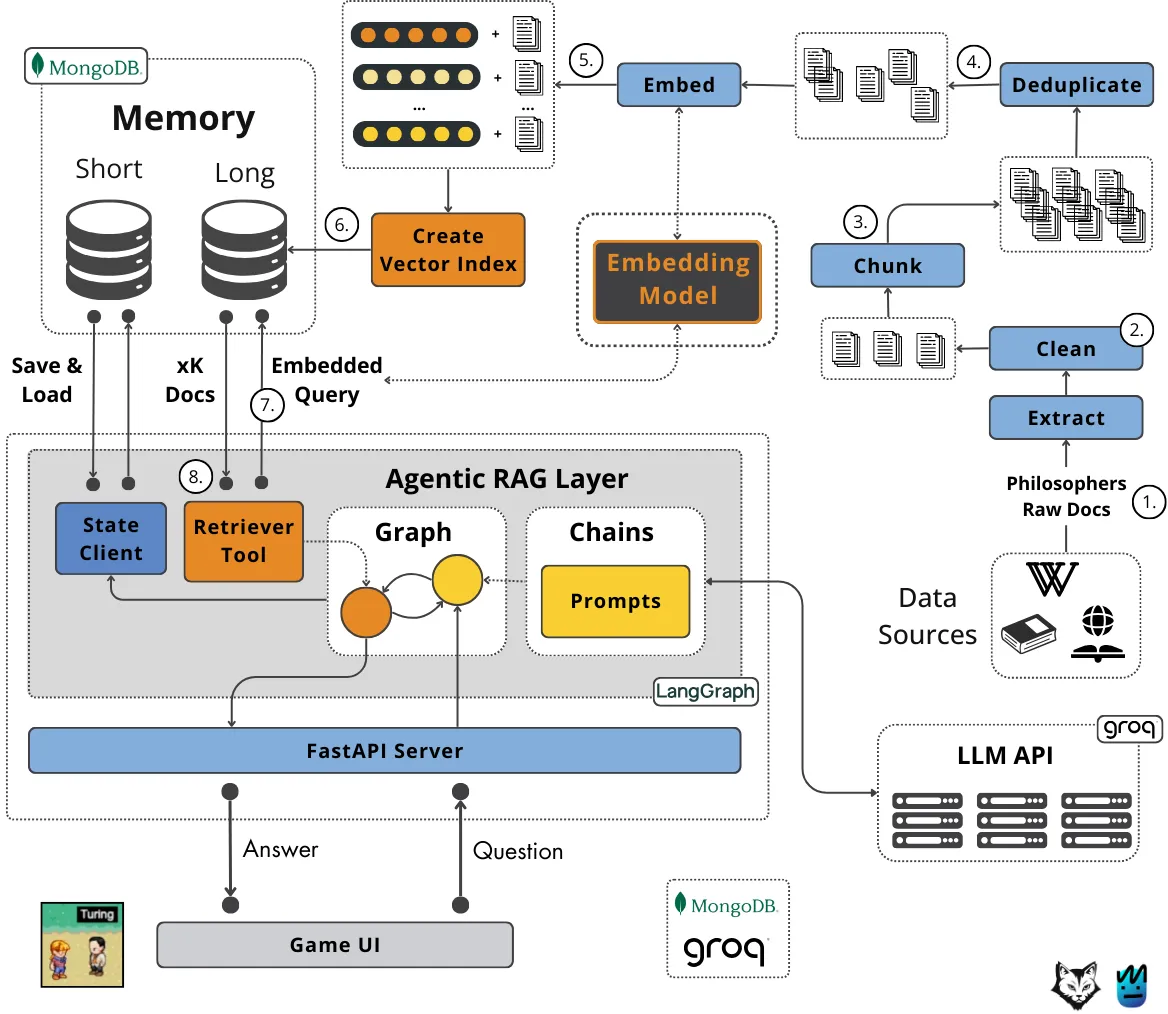
How "Memory Files" Work:
"Memory files" are essentially structured data stores that Claude 4 can use to:
- Persist Key Information: Store important facts,user preferences,project details,summaries of previous interactions,or intermediate results from long computations.
- Maintain Context Across Sessions: If a user starts a project with Claude 4 today and continues tomorrow,the model can load relevant information from a memory file to "remember" where they left off,what decisions were made,and what the overall goals are.
- Handle Information Exceeding Context Window: For projects or knowledge bases far larger than 200K tokens,memory files can serve as an external knowledge source that Claude 4 learns to query and update strategically.
- Structured Learning: The model can be trained or prompted to organize information within these files in a structured way (e.g.,key-value pairs,summaries,timelines),making retrieval more efficient.
The Files API is the mechanism enabling this. Users would grant Claude 4 permission to read from and write to specific files or directories. The model then uses its intelligence to decide what information is worth "remembering" (writing to a file) and when to retrieve information from these files to inform its current task.
Technical Implementation Considerations (Speculative):
The exact format of these memory files isn't detailed,but they could range from simple text files or JSON/YAML structures to more sophisticated,model-optimized formats. The key is the model's ability to intelligently manage these external stores. This likely involves:
- Retrieval-Augmented Generation (RAG) on Steroids: While RAG typically involves retrieving from static vector databases,Claude 4's memory files suggest a more dynamic read/write interaction with evolving external knowledge.
- Learned Memory Management Policies: The model might be trained to learn optimal strategies for when to save,what to save,how to summarize,and when to retrieve information to balance detail with efficiency.
- Summarization and Abstraction: To avoid memory files becoming unwieldy,Claude 4 might employ summarization techniques to condense information before storing it.
Benefits and Use Cases:
- Long-Duration Autonomous Tasks: Essential for the 7+ hour autonomous work capability. An agent working on a large software project can store its progress,plans,encountered issues,and solutions in memory files,allowing it to "sleep" and resume effectively.
- Personalized AI Assistants: An assistant could maintain a memory file of a user's preferences,past requests,and ongoing projects,leading to highly personalized and context-aware interactions over time.
- Complex Research Projects: Researchers can use Claude 4 to incrementally build a knowledge base in memory files,adding findings,notes,and hypotheses as the research progresses over weeks or months.
- Multi-Session Collaborative Work: Teams collaborating with an AI agent on a design or document can rely on memory files to ensure the AI retains shared context and decisions across different users and interaction times.
Security and Persistence Implications:
The use of memory files introduces important considerations:
- Data Security: Since memory files reside on user-controlled storage,users are responsible for securing these files. Sensitive information stored by the AI needs appropriate protection.
- Data Privacy: Users must be aware of what information the AI is storing and have control over it. Clear consent and management mechanisms are vital.
- Persistence: Information in memory files persists beyond the current session,unlike in-context memory which is lost when the session ends. This is a powerful feature but requires careful management of stored data.
- Access Control: Proper file permissions and access controls are necessary to prevent unauthorized access to or modification of memory files,especially in multi-user or enterprise settings.
Enhanced Memory with file capability is a crucial step towards AI systems that can learn and operate effectively over truly long timescales,overcoming the limitations of purely ephemeral context windows and enabling more robust and intelligent agentic behavior.
3.4 Massive 200K+ Token Context Window:Processing Vast Information Scopes
Anthropic has long been a leader in providing models with large context windows,and Claude 4 continues this tradition by offering a **context window exceeding 200,000 tokens** for both Opus 4 and Sonnet 4. This substantial capacity allows the models to process and reason over incredibly large amounts of text in a single pass.
What Does 200K Tokens Mean in Practice?
To put this into perspective,200,000 tokens can roughly equate to:
- Approximately **150,000 words** (since 1 token is about 0.75 words on average for English).
- A very long book (e.g.,"Moby Dick" is around 200,000 words,"The Great Gatsby" is about 50,000 words). So,Claude 4 can ingest and analyze one very long novel or several shorter ones simultaneously.
- Many thousands of lines of code. A large software module or even a small-to-medium sized codebase might fit within this context.
- Extensive legal documents,lengthy financial reports,or comprehensive research papers.
Benefits and Applications:
The ability to "see" and process such a vast amount of information at once unlocks numerous capabilities:
- Deep Document Comprehension and Synthesis: Claude 4 can read an entire annual report and answer detailed questions,summarize key findings from a long research paper,or analyze complex legal contracts for specific clauses and implications without losing track of earlier parts of the document.
- Full Codebase Analysis: Developers can feed large sections of a codebase to Claude 4 to ask for explanations,identify potential bugs that span multiple files,suggest refactoring opportunities,or ensure consistency across modules.
- Long-Form Content Generation: When writing extensive reports,articles,or even book chapters,Claude 4 can maintain coherence and thematic consistency over much longer stretches of text.
- Enhanced Conversational Context: In extended dialogues,the model can remember details and nuances from much earlier in the conversation,leading to more natural,relevant,and less repetitive interactions.
- Complex Q&A over Large Datasets: Users can provide large textual datasets (e.g.,product reviews,customer feedback transcripts) and ask Claude 4 to extract insights,identify trends,or answer specific questions based on the full dataset.
- "Needle in a Haystack" Tasks: Anthropic has previously demonstrated that its models with large context windows perform well at accurately recalling specific pieces of information embedded within very long documents.
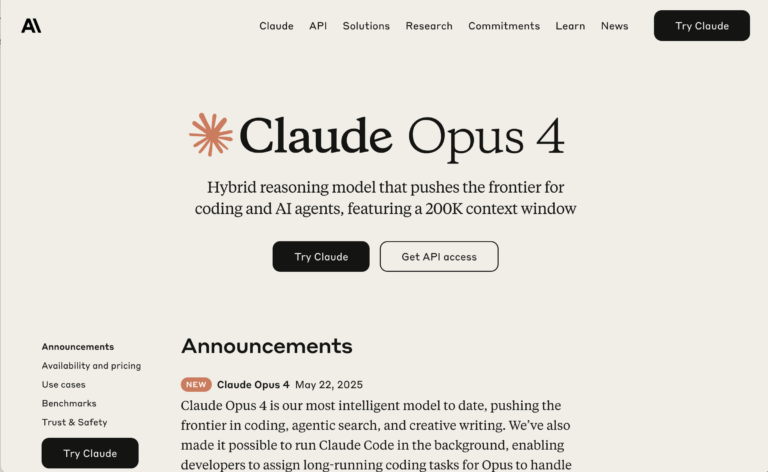
While other models are also pushing context window sizes (e.g.,Google Gemini 1.5 Pro with up to 1 million tokens),a 200K window is still exceptionally large and highly practical for a vast majority of real-world use cases. The key is not just the size,but the model's ability to effectively *utilize* that context,maintain attention across the entire length,and accurately retrieve information,areas where Anthropic models have traditionally performed well.
Combined with the "Enhanced Memory" file capabilities,the 200K context window provides Claude 4 with a powerful two-tiered approach to information management:a large "working memory" for immediate tasks and a persistent "long-term memory" for ongoing projects and knowledge retention.
3.5 Advanced Coding & Reasoning,Featuring Claude Code
A central pillar of Claude 4's design is its significantly enhanced proficiency in **coding and logical reasoning**. Anthropic positions Claude Opus 4 as potentially "the best coding model in the world," a claim backed by strong benchmark performances (discussed in detail later) and new developer-focused tools like **Claude Code**.
Elevated Coding Prowess:
Claude 4's coding capabilities extend far beyond simple snippet generation:
- Complex Algorithm Implementation: Generating efficient and correct code for complex algorithms and data structures.
- Bug Detection and Fixing: Identifying subtle bugs in existing codebases (leveraging its large context window) and proposing accurate fixes. This is a key aspect tested by benchmarks like SWE-bench.
- Code Refactoring and Optimization: Suggesting improvements to code for readability,performance,or maintainability.
- Test Generation: Automatically creating unit tests and integration tests for given code.
- Code Explanation and Documentation: Understanding complex code and generating clear explanations or documentation for it.
- Multi-File Project Understanding: Reasoning about dependencies and interactions across multiple files in a software project.
Example:Complex Problem-Solving Walkthrough (Hypothetical)
Consider a developer tasking Claude Opus 4:
"I have a microservices architecture with services A,B,and C. Service A calls B,which then calls C. We're seeing intermittent high latency in requests to A. The logs show that sometimes service C takes over 500ms to respond to B. However,C's own metrics show its internal processing time is usually under 50ms. The services communicate via gRPC with retries. Here are the relevant code snippets for the client in B calling C,and the server endpoint in C. Can you help diagnose potential issues and suggest improvements?"
Claude Opus 4,leveraging its advanced reasoning and coding understanding,might proceed as follows:
- Context Ingestion: Analyze the provided code for gRPC client/server setup,retry logic,timeouts,and any specific library usage.
- Hypothesis Generation (Extended Thinking):
- Network latency between B and C.
- Inefficient serialization/deserialization.
- Retry storm issues if timeouts are misconfigured.
- Connection pooling problems in B's gRPC client.
- Resource contention on the host running C,affecting network stack or gRPC request handling before internal processing.
- Issues with load balancing if multiple instances of C exist.
- Code Analysis & Questioning: "Looking at B's client code,the timeout for calls to C is set to 600ms,and retries are configured for up to 3 attempts. Could there be scenarios where multiple retries stack up,leading to the perceived latency in A,especially if C is occasionally slow for reasons external to its core logic (e.g.,network blip,GC pause)?"
- Suggesting Debugging Steps/Code Modifications: "To isolate this,I recommend adding more detailed logging in B around each call attempt to C,including timestamps before call,after response,and for any errors. Also,consider implementing a circuit breaker pattern in B. For C,while internal processing is fast,ensure that request queuing or thread pool exhaustion isn't an issue at the gRPC server level under load. Here's an example of how you might add more granular timing logs..." (Provides modified code snippets).
This demonstrates reasoning beyond simple code generation,incorporating architectural understanding and diagnostic thinking.
Claude Code:The Developer's Integrated Co-pilot
To directly embed these advanced coding capabilities into developer workflows,Anthropic has made **Claude Code** generally available. This is a specialized offering that includes:
- Native IDE Integrations: Claude Code offers plugins for popular Integrated Development Environments (IDEs) like **VS Code** and **JetBrains IDEs** (IntelliJ IDEA,PyCharm,etc.). This allows developers to interact with Claude 4 directly within their coding environment.
- Inline Code Edits and Suggestions: Developers can highlight code,ask questions,request refactoring,or get suggestions for completing code blocks,all without leaving their IDE.
- Debugging Assistance: Help identify and fix bugs by providing context and code to Claude Code.
- SDK for Custom Agents: Anthropic provides an SDK (Software Development Kit) enabling developers to build custom AI agents powered by Claude 4. These agents can be tailored for specific development tasks,team workflows,or proprietary codebase analysis.
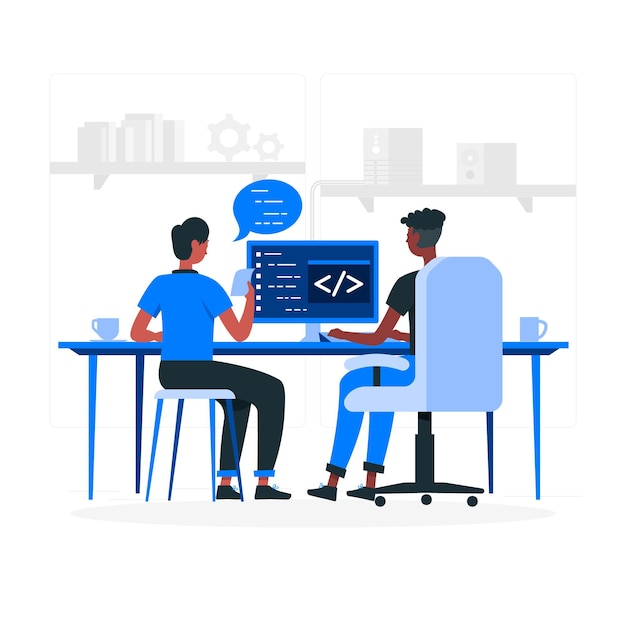
Enhanced Logical Reasoning:
Beyond coding,Claude 4 exhibits stronger general logical reasoning,crucial for:
- Mathematical Problem Solving: As seen in improved AIME benchmark scores.
- Scientific Reasoning: Tackling graduate-level questions (GPQA Diamond).
- Complex Planning and Strategy: Devising multi-step plans for agentic tasks.
- Understanding Nuance and Ambiguity: Better interpretation of complex prompts and inferring user intent.
This combination of elite coding skills,integrated developer tools,and robust logical reasoning makes Claude 4 a formidable asset for technical problem-solving and software innovation.
3.6 Reduced Shortcut Behavior:Enhancing Reliability in Agentic Tasks
A critical challenge in developing capable AI agents is their tendency to find "shortcuts" or "loopholes" to achieve a stated goal without performing the task robustly or as intended. Anthropic reports a significant **65% reduction in this shortcut behavior** for Claude 4 models compared to its predecessor,Sonnet 3.7,on susceptible agentic tasks. This is a vital improvement for building trustworthy and reliable AI systems.
Understanding Shortcut Behavior:
Shortcut behavior,sometimes called "reward hacking" in reinforcement learning contexts,occurs when an AI model optimizes for a narrowly defined objective in an unexpected or undesirable way. Examples include:
- Superficial Answers: Providing a brief or incomplete answer that technically satisfies the prompt but lacks depth or thoroughness,especially for difficult questions.
- Exploiting Evaluation Flaws: If an agent is evaluated on a simple metric (e.g.,"file exists"),it might create an empty file instead of correctly generating the file's content.
- Avoiding Difficult Steps: If a multi-step task has a complex intermediate step,the agent might try to bypass it or perform it poorly if it can still achieve a partial goal.
- Over-reliance on Simple Tools: An agent might repeatedly use a basic web search for every piece of information,even when more sophisticated reasoning or internal knowledge would be more appropriate and efficient.
How Claude 4 Mitigates Shortcuts:
The 65% reduction likely stems from several factors in Anthropic's training methodology,rooted in their Constitutional AI approach and ongoing research into agent alignment:
- Refined Training Objectives: Training goals that penalize superficial or incomplete solutions and reward thorough,multi-step reasoning and correct tool usage. This might involve more sophisticated reward models in Reinforcement Learning from Human Feedback (RLHF) or AI Feedback (RLAIF).
- Adversarial Training: Exposing the model during training to prompts and scenarios specifically designed to elicit shortcut behaviors,and then teaching it to avoid them.
- Constitutional AI Principles: The underlying principles guiding the model's behavior likely include directives promoting thoroughness,honesty,and helpfulness,which inherently discourage shortcuts.
- Improved "Extended Thinking": The ability to engage in deeper,more deliberative reasoning (as discussed in Hybrid Reasoning) naturally makes the model less reliant on quick,superficial solutions.
- Better Evaluation Metrics during Training: Anthropic likely uses more nuanced internal benchmarks and evaluation criteria during development that are harder to "game" than simpler metrics. The 65% reduction itself implies a quantifiable metric for assessing this behavior.
Impact on Reliability and Trustworthiness:
- More Robust Agents: AI agents built with Claude 4 are more likely to perform tasks correctly and completely,even complex ones,rather than finding undesirable workarounds.
- Increased Predictability: Reduced shortcutting leads to more predictable and dependable behavior,which is crucial for deploying AI in mission-critical applications.
- Greater User Trust: Users are more likely to trust and rely on an AI system that consistently demonstrates thoroughness and avoids "cheating" on tasks.
- Safer AI Systems: In some contexts,shortcut behavior could lead to unsafe outcomes if an agent bypasses critical safety checks or procedures. Reducing this tendency is a step towards safer AI.
The significant reduction in shortcut behavior is a testament to Anthropic's focus on not just raw capability,but also on the qualitative aspects of AI performance that are essential for real-world utility and safety. It makes Claude 4 a more dependable foundation for building the sophisticated autonomous systems of the future.
4. Technical Architecture Deep Dive:Unpacking Claude 4's Inner Workings
While Anthropic,like other leading AI labs,maintains a degree of secrecy around the precise architectural details and training datasets of its flagship models,the announced features,performance characteristics,and broader trends in AI research allow us to make educated inferences about the technical underpinnings of Claude 4. This section explores the likely innovations and architectural concepts that enable its advanced capabilities.
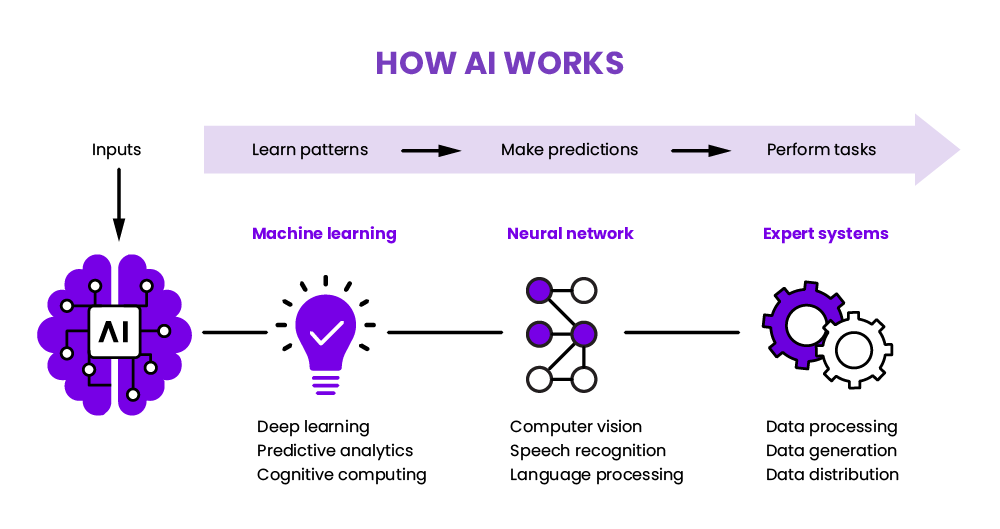
4.1 Beyond Standard Transformers:Evolving the Core Architecture
It's highly probable that Claude 4 is built upon an advanced iteration of the Transformer architecture,which has been the dominant paradigm for large language models. However,to achieve features like a 200K context window,hybrid reasoning,and efficient parallel tool use,Anthropic has likely incorporated several significant modifications and extensions:
- Attention Mechanism Enhancements: Standard Transformer attention mechanisms have quadratic complexity with sequence length,making them computationally prohibitive for very long contexts like 200K tokens. Anthropic likely employs advanced sparse attention variants (e.g.,Longformer,BigBird-style),FlashAttention-like optimizations for I/O efficiency,or perhaps hierarchical attention mechanisms that process information at different granularities. These techniques aim to capture long-range dependencies without incurring unmanageable computational costs.
- Mixture of Experts (MoE): Some large models leverage MoE layers,where different parts of the input are routed to specialized sub-networks ("experts"). This can increase model capacity and specialization while keeping computational cost per token relatively controlled during inference. While not explicitly confirmed for Claude 4,it's a plausible technique for scaling capabilities.
- Optimized Positional Encodings: Effective methods for encoding token positions are crucial for long sequences. Anthropic might be using relative positional encodings (like RoPE,used in Llama) or other advanced techniques that scale better to long contexts than original absolute positional encodings.
- Improved Normalization and Activation Functions: Ongoing research continually refines these core components for better training stability,faster convergence,and improved performance. Claude 4 would incorporate the latest best practices or novel approaches.
4.2 Orchestrating Hybrid Reasoning:The "Dual-Process" System
The "Hybrid Reasoning" feature,enabling both "Instant" and "Extended Thinking" modes,suggests a sophisticated control mechanism or even a composite architecture. This could be conceptualized as an AI parallel to human "System 1" (fast,intuitive) and "System 2" (slow,deliberate) thinking:
- Fast Pathway (Instant Mode): A highly optimized part of the network,or a smaller,specialized sub-model,trained for rapid responses to common queries and simpler tasks. This pathway would prioritize low latency and efficiency.
- Deliberative Pathway (Extended Thinking): A more computationally intensive pathway,potentially involving more layers,more attention heads,or explicit iterative reasoning loops (internal "scratchpad" or chain-of-thought). This pathway would be invoked when the model's internal confidence for a quick answer is low,or when the task complexity,detected from the prompt,necessitates deeper analysis.
- Orchestration Layer/Router: A crucial component would be a learned mechanism that decides which pathway to engage,or how to blend their outputs. This router might analyze prompt complexity,query type,or even use initial,fast pathway outputs to determine if "Extended Thinking" is required. This is where the model decides to use tools or delve deeper.
- Resource Management: The "Extended Thinking" mode implies dynamic resource allocation. The system needs to manage the increased computational load associated with deeper reasoning,potentially impacting token generation speed or cost.
This hybrid approach allows Claude 4 to optimize for both speed on simple tasks and accuracy/thoroughness on complex ones,a significant step towards more versatile and practical AI.
4.3 Enabling Parallel Tool Use:An Advanced Agentic Framework
Parallel tool use is a hallmark of advanced agentic capabilities. Architecturally,this requires more than just sequential function calling. It implies:
- Multi-threaded Planning and Execution: The model must be able to identify parts of a task that can be parallelized and generate a plan that includes concurrent tool invocations.
- Asynchronous Operation Management: An internal system to initiate multiple tool calls (e.g.,API requests,code execution threads) and manage their asynchronous responses. This involves tracking pending operations,handling timeouts or errors for individual calls,and collecting results as they become available.
- State Management for Concurrent Tasks: Maintaining context and state for each parallel branch of execution.
- Sophisticated Result Synthesis: Once parallel operations complete,the model needs to integrate the (potentially diverse) information from multiple tool outputs into a coherent final response or subsequent action plan. This might involve resolving conflicts or inconsistencies between tool outputs.
- Tool Registry and Invocation Mechanism: A robust system for defining available tools (like the Code Interpreter,MCP Connector,Files API),their input/output schemas,and securely invoking them. The MCP (Multi-Capability Platform) Connector sounds like a generalized interface for diverse enterprise tools.
This capability likely involves a specialized agentic loop or framework built around the core LLM,allowing it to act more like an orchestrator of multiple processes rather than just a linear text generator.

4.4 Enhanced Memory & File Access:Structuring Long-Term Knowledge
The combination of a 200K token context window and "memory files" provides a powerful hierarchical memory system:
- In-Context Memory (200K Tokens): This acts as the model's "working memory" or "short-term memory," allowing it to hold and process a vast amount of information relevant to the current interaction. Efficiently utilizing this large context likely involves techniques to prevent "lost in the middle" problems,ensuring the model attends to all parts of the input.
- Externalized Memory (Memory Files via Files API): This represents a form of "long-term memory" that is persistent and can be explicitly managed. Architecturally,this requires:
- A Learned Read/Write Policy: The model must learn *what* information is critical to save to a memory file,*when* to save it,and *how* to retrieve relevant information from these files when needed. This could be part of its RLHF/RLAIF training.
- Structured Data Handling: While the exact format isn't specified,the model might learn to structure information in these files (e.g.,as summaries,key facts,decision logs) to facilitate efficient retrieval and updating.
- Integration with Tool Use: The Files API is a tool. The decision to read from or write to a memory file is an action the agentic framework can take,similar to calling any other tool.
- Security and Sandboxing: The Files API interactions must be carefully sandboxed to prevent unauthorized file access,ensuring the model only interacts with permitted files/directories.
This system moves beyond simple RAG by allowing the model to *dynamically create and modify* its external knowledge base,essential for learning and adaptation over long-duration tasks.
4.5 Training Data and Methodologies:The Unseen Foundation
The capabilities of Claude 4 are heavily dependent on the quality and scale of its training data and the sophistication of its training methodologies:
- Vast and Diverse Datasets: Training likely involved petabytes of text and code data,carefully curated for quality,diversity,and to minimize harmful biases. Specialized datasets for coding,reasoning,and dialogue would have been crucial.
- Advanced Self-Supervised Learning: Beyond standard next-token prediction,Anthropic might employ more advanced self-supervised objectives to foster deeper understanding.
- Reinforcement Learning from AI Feedback (RLAIF) / Constitutional AI: This is a hallmark of Anthropic's approach. Instead of relying solely on human labelers for RLHF,Anthropic uses AI models to help supervise other AI models,guided by a "constitution" of principles. This allows for scaling up preference data generation and instilling desired behaviors (like harmlessness,honesty,and reducing shortcuts) more effectively. The 65% reduction in shortcut behavior is a likely outcome of these advanced alignment techniques.
- Curriculum Learning & Specialized Fine-Tuning: The model might have undergone stages of training,starting with general knowledge and then being progressively fine-tuned on more specialized tasks like coding,complex reasoning,and tool use.
- Data for Long Context: Specific training strategies are needed to teach models to effectively use very long context windows,ensuring they can cohere information and answer questions based on text spanning hundreds of thousands of tokens.
4.6 Computational Costs,Efficiency,and Trade-offs
Achieving SOTA performance with models like Claude 4,especially Opus 4 in its "High Compute" modes,involves significant computational resources for both training and inference:
- Training Costs: Training these models likely requires thousands of high-end GPUs/TPUs running for weeks or months,representing a massive upfront investment.
- Inference Costs: Opus 4 is positioned as a premium model,implying higher inference costs per token or per query compared to Sonnet 4 or previous generation models. The "High Compute" mode for benchmarks like SWE-bench (involving parallel attempts and patch rejection) would be particularly resource-intensive.
- Latency vs. Capability: The "Hybrid Reasoning" modes explicitly acknowledge this trade-off. "Instant" mode prioritizes low latency,while "Extended Thinking" prioritizes capability at the cost of potentially higher latency and compute.
- Model Quantization and Optimization: Anthropic likely employs various techniques (e.g.,quantization,optimized kernels,efficient serving infrastructure) to make inference as efficient as possible without unduly sacrificing performance,especially for the more cost-sensitive Sonnet 4.
- The Sonnet 4 / Opus 4 Split: This dual-model strategy itself is a way to manage the cost-capability trade-off,offering users a choice based on their specific needs and budget. Sonnet 4 aims to provide a large fraction of Opus 4's capability at a significantly lower operational cost.
Understanding these computational aspects is crucial for enterprises planning to deploy Claude 4 at scale,as they will need to factor in the cost implications of using these powerful models,particularly for high-volume or latency-sensitive applications.
A Note on Speculation: It's important to reiterate that many architectural details are proprietary. The discussion above is based on publicly announced features,performance claims,and prevailing trends in AI research. The true innovations within Claude 4 might be even more nuanced or novel than what can be inferred externally.
5. Performance Benchmarks Analysis:Quantifying Claude 4's Prowess
The launch of any major AI model is accompanied by a suite of benchmark results,providing quantitative measures of its capabilities against established tasks and,implicitly,against its competitors. Claude 4,particularly the flagship Opus 4,has posted impressive scores across several critical benchmarks,establishing new state-of-the-art (SOTA) results in areas vital for coding,reasoning,and autonomous agentic functions. This section delves into these benchmarks,explaining their significance and what Claude 4's performance truly signifies.
It's important to note that benchmarks,while useful,provide only a partial view of a model's overall utility. Real-world performance can vary based on specific use cases,prompt engineering,and integration. Anthropic also distinguishes between "Standard" performance and "High Compute" performance for some benchmarks with Opus 4,where "High Compute" involves techniques like parallel attempts and patch rejection,trading more compute for higher scores.
5.1 Comprehensive Benchmark Results Overview (Claude Opus 4 & Sonnet 4)
The following table summarizes key benchmark scores reported for Claude 4,with comparisons to approximate previous SOTA where available,largely based on figures from the provided synthesis document. Note that "Previous SOTA" can be a moving target and often refers to models like GPT-4 series or Gemini.
Benchmark | Description & Significance | Claude Opus 4 (Standard) | Claude Opus 4 (High Compute) | Claude Sonnet 4 (Standard) | Approx. Previous SOTA (e.g.,GPT-4,Gemini) |
---|---|---|---|---|---|
SWE-bench (repo-level) | Fixing real-world GitHub issues in Python repositories. Tests deep code understanding,debugging,and patch generation. Highly indicative of practical software engineering skill. | 72.5% | 79.4% | 72.7% | ~65-70% |
Terminal-bench | Autonomous terminal operations,file system navigation,and file editing to achieve goals. Directly measures agentic capability in a simulated OS environment. | 43.2% | 80.2% | N/A (Opus 4 focused) | Significantly Lower (Historically) |
MMLU | Massive Multitask Language Understanding. 57 diverse subjects (humanities,STEM,social sciences). Measures broad knowledge and reasoning. | 87.4% | N/A | Competitive (Slightly lower than Opus) | ~86-88% (e.g. GPT-4) |
GPQA Diamond | Graduate-level Google-Proof Q&A. Difficult reasoning questions designed to be hard for web search. Tests deep expert-level reasoning. | 74.9% | N/A | Competitive | ~60-70% |
HumanEval (Python) | Code generation benchmark. Synthesizing Python functions from docstrings. Measures basic to intermediate coding proficiency. | ~85% (Est.) | N/A | Competitive | ~75-80% (GPT-4 Est.) |
AIME Problems | American Invitational Mathematics Examination. Challenging high-school level math competition problems. Tests mathematical reasoning and problem-solving. | 33.9% | N/A | Competitive | ~25-30% |
MMMU | Massive Multi-discipline Multimodal Understanding. Tests understanding across various modalities and disciplines. Claude 4 tested on text-based components. | 73.7% | N/A | Competitive | Varies (Higher for native multimodal models like Gemini Ultra on full test) |
Key Takeaways from Benchmark Performance:
- Dominance in Coding and Agentic Tasks: Claude Opus 4,especially in "High Compute" mode,sets new SOTA records on SWE-bench and Terminal-bench. This strongly supports Anthropic's claims of leadership in practical software engineering and autonomous agent capabilities.
- Sonnet 4's Impressive SWE-bench Score: Claude Sonnet 4 matching or slightly exceeding Opus 4 (Standard) on SWE-bench (72.7% vs 72.5%) is remarkable. It suggests Sonnet 4 is highly optimized for coding tasks and offers exceptional value for such applications.
- Strong General Reasoning: High scores on MMLU,GPQA Diamond,and AIME confirm that Claude 4 possesses robust general knowledge and advanced logical and mathematical reasoning skills,competitive with or exceeding other top models.
- "High Compute" Significance: The substantial performance lift for Opus 4 in "High Compute" mode on SWE-bench and Terminal-bench indicates a deep well of capability that can be unlocked with additional computational resources. This suggests that the model's architecture can support more intensive,deliberative processing when required.
- Balanced Performance: While excelling in coding,Claude 4 maintains strong performance across a broad range of reasoning tasks,making it a versatile model.
5.2 Deep Dive:Coding Excellence & SWE-bench Leadership
The **SWE-bench (Software Engineering Benchmark)** is arguably one of the most challenging and realistic benchmarks for evaluating an AI's coding capabilities. It involves resolving actual bugs and feature requests sourced from open-source GitHub repositories. Success requires not just generating code,but understanding existing complex codebases,accurately diagnosing issues,formulating correct patches,and often interacting with build and test environments.
The near SOTA performance of Standard Sonnet 4 (72.7%) is particularly noteworthy,indicating it is a highly capable and potentially more cost-effective option for many demanding coding tasks. Opus 4's 72.5% in Standard mode is already excellent,but its jump to **79.4% with "High Compute"** (involving strategies like parallel attempts and patch rejection) firmly establishes a new industry benchmark for automated software engineering. This suggests Opus 4 can,with sufficient resources,tackle even more challenging coding problems that were previously out of reach for AI. This level of performance has profound implications for developer productivity,potentially automating significant portions of debugging and maintenance work.
5.3 Deep Dive:Autonomous Terminal Operations (Terminal-bench)
**Terminal-bench** is a benchmark specifically designed to evaluate an AI's proficiency in operating autonomously within a command-line (shell) environment. This involves understanding natural language instructions,translating them into sequences of bash commands,navigating file systems,creating/editing files,running scripts,and verifying outcomes. It's a direct test of an AI's ability to act as an autonomous agent in a complex,stateful environment.
Terminal-bench Performance (Claude Opus 4)
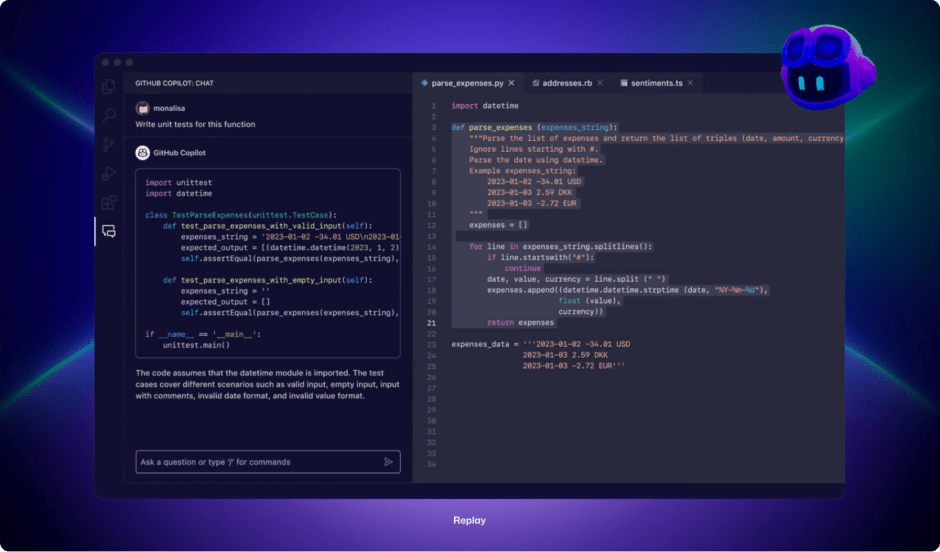
The performance of Claude Opus 4 on Terminal-bench is particularly striking. While the "Standard" score of 43.2% is already competent,the leap to **80.2% in "High Compute" mode is a watershed moment for agentic AI**. It indicates that with adequate computational budget and sophisticated execution strategies,Opus 4 can reliably plan and execute complex sequences of actions in a simulated operating system. This capability is fundamental for building AI agents that can perform system administration,automated software deployment,data processing pipelines,or assist with complex development operations. An 80.2% success rate on such a challenging benchmark sets a very high bar for autonomous interaction and task completion in digital environments.
5.4 Deep Dive:Expanded Reasoning Capabilities
Beyond its specialized coding and agentic prowess,Claude 4 demonstrates substantial gains in core reasoning and knowledge application,as evidenced by its performance on benchmarks like MMLU,GPQA Diamond,and AIME. These benchmarks probe a model's ability to recall information,apply logical deduction,and solve complex problems across diverse academic and scientific fields.
MMLU
Massive Multitask Language Understanding:Assesses knowledge and reasoning across 57 subjects (e.g.,history,law,math,ethics,computer science).
Opus 4 Score:87.4%. Demonstrates broad,expert-level general knowledge and problem-solving ability.
GPQA Diamond
Graduate-Level Google-Proof Q&A:Focuses on complex scientific and expert-level reasoning questions that are difficult to answer using simple web searches.
Opus 4 Score:74.9%. Indicates very strong capabilities in deep reasoning and understanding complex,nuanced expert topics.
AIME Problems
American Invitational Mathematics Examination:Involves challenging multi-step mathematical problems requiring significant logical deduction and problem-solving skills.
Opus 4 Score:33.9%. A strong score on AIME reflects advanced mathematical reasoning capabilities,a traditionally difficult area for LLMs.
MMMU (Text Components)
Massive Multi-discipline Multimodal Understanding:Evaluates understanding across various data types and subjects. Claude 4's score reflects its performance on text-based portions.
Opus 4 Score:73.7%. Shows strong multidisciplinary textual understanding,though not fully comparable to native multimodal models on the full benchmark.
Significance of Reasoning Benchmark Scores:
A high MMLU score (87.4% for Opus 4) signifies a vast and accurately recallable knowledge base,approaching or exceeding human expert performance in many of the tested domains. This is foundational for any advanced AI.
However,excelling on benchmarks like GPQA Diamond (74.9%) and AIME (33.9%) is arguably more indicative of true reasoning depth. GPQA questions are designed to resist simple retrieval from web search,demanding genuine understanding and inference. AIME problems require multi-step logical deduction and application of mathematical concepts. Claude 4's strong performance here,particularly the SOTA score on GPQA Diamond,suggests a qualitative improvement in its ability to perform complex,analytical "thought" processes.
These advanced reasoning capabilities,combined with the reduced shortcut behavior,underpin Claude 4's "Extended Thinking" mode. They are crucial for tasks requiring sophisticated analysis,research synthesis,strategic planning,and complex decision-making in agentic scenarios. While Opus 4's scores on MMMU (73.7%) and GPQA Diamond (74.9%) are high,it's noted in some analyses (referenced in review documents) that these specific scores might not surpass those of the prior Claude 3 Opus model,indicating that progress isn't always linear across every single metric for every new model generation,and that SOTA can be nuanced. However,the overall package of Claude 4,especially in coding and agentic benchmarks,shows clear advancement.
6. Competitive Landscape Analysis:Claude 4 vs. Other Leading AI Models
The field of large language models is intensely competitive,with major players like OpenAI (with its GPT series,including the recent GPT-4o),Google (with its Gemini family),and others constantly pushing the boundaries of AI capability. Claude 4,with Opus 4 and Sonnet 4,enters this dynamic arena not merely as a participant but as a formidable contender,establishing clear leadership in several key areas while offering competitive performance across others. This section provides a detailed comparison based on available information and benchmark results.
6.1 Comprehensive Feature & Performance Matrix
This table aims to provide a feature-by-feature and benchmark-based comparison between Claude 4 models and their primary competitors. Note that competitor performance can vary by specific version and benchmark run;figures are approximate and aim to reflect the general competitive positioning at the time of Claude 4's launch.
Feature / Benchmark Parameter | Claude Opus 4 | Claude Sonnet 4 | OpenAI GPT-4o / GPT-4 Turbo | Google Gemini 1.5 Pro / Ultra |
---|---|---|---|---|
SWE-bench (Peak/High Compute) | 79.4% (Leader) | 72.7% (Standard) (Strong) | ~65-70% (Est.) | ~60-70% (Est.) |
Terminal-bench (Peak/High Compute) | 80.2% (Leader) | N/A (Opus Focused) | Significantly Lower | Lower / Not Directly Comparable |
Autonomous Work Duration (Validated) | 7+ Hours | Extended (Hours) | Limited (Typically shorter sessions for similar complexity) | Context-dependent,less focus on sustained agentic work |
Context Window (Tokens) | 200K+ | 200K+ | 128K (GPT-4 Turbo/4o) | Up to 1 Million (Gemini 1.5 Pro) (Leader in size) |
Parallel Tool Use | ✓(Advanced) | ✓(Advanced) | ~(Emerging/Sequential focus) | ~(Function calling,improving) |
Enhanced Memory (Persistent File Access) | ✓(Native,Structured) | ✓(Native,Structured) | ~(Via RAG/Plugins,less integrated) | ~(Via RAG/External,less integrated) |
Reduced Shortcut Behavior (vs. Prior Gen) | 65% Reduction | 65% Reduction | Improving,Varies | Improving,Varies |
MMLU Score (General Reasoning) | 87.4% | Competitive | ~86-88% (Highly Competitive) | Highly Competitive (Gemini Ultra) |
GPQA Diamond (Expert Reasoning) | 74.9% (Leader) | Competitive | Lower (Est.) | Lower (Est.) |
Multimodal Capabilities (Vision,Audio) | ✗(Text-focused at launch,planned) | ✗(Text-focused at launch,planned) | ✓(Strong,esp. GPT-4o) | ✓(Strong,native multimodal) |
Pricing Tier (Relative) | Premium / Highest | Mid-Range / High Value | Varies (GPT-4o competitive,Turbo higher) | Varies (1.5 Pro competitive for context) |
Primary Safety Framework | Constitutional AI,ASL-3 | Constitutional AI,ASL-3 | Safety Filters,Moderation API,RLHF | Responsible AI Toolkit,Safety Filters |
6.2 Qualitative Deep Dive:Where Claude 4 Excels and Faces Challenges
Unmatched Strengths:Coding,Agentic Workflows,and Sustained Task Execution
Claude 4,particularly Opus 4,carves out a clear leadership position in tasks demanding sophisticated **software engineering skills and autonomous agentic behavior**. Its SOTA performance on SWE-bench and Terminal-bench is not just incremental;it represents a qualitative leap. While GPT-4 and Gemini are highly capable coders for many tasks,Claude 4's ability to resolve complex,real-world GitHub issues and operate autonomously in a terminal environment for extended periods (7+ hours validated for Opus 4) suggests a deeper,more robust understanding of code semantics,execution environments,and multi-step problem-solving.
The combination of **Hybrid Reasoning,advanced Parallel Tool Use,and Enhanced Memory (with file access)** provides an architectural advantage for building complex,long-running agents. Competitors often rely on more sequential tool use or less integrated memory solutions (e.g.,RAG with external vector stores). Claude 4's native ability to manage parallel operations and maintain persistent,structured memory across sessions gives it an edge for use cases like:
- Automated debugging and code maintenance cycles that span hours.
- Complex research tasks involving gathering data from multiple sources,analyzing it,and iteratively refining hypotheses over days.
- Sophisticated business process automation that requires interaction with multiple enterprise systems and adaptation to dynamic conditions.
Context Window and Information Processing:A Competitive Arena
In terms of raw context window size,Google's Gemini 1.5 Pro currently leads with its capability of up to 1 million tokens. Claude 4's 200K+ token window is still exceptionally large and highly practical for the vast majority of tasks. More critically,the effectiveness of a large context window depends on the model's ability to reliably retrieve and reason over information across that entire span (the "needle in a haystack" problem) and avoid performance degradation. Anthropic models have historically demonstrated strong performance in long-context recall.
Furthermore,Claude 4's **Enhanced Memory file capability** offers a complementary approach to handling information that exceeds even its large context window or needs to persist across sessions. This structured,persistent memory,which the model can actively manage,may offer advantages in reliability and coherence for extremely long or complex analytical tasks compared to solely relying on an ultra-long,ephemeral context window. The optimal balance between massive in-context memory and structured external memory is an active area of research and development across the industry.
General Reasoning and Knowledge:Top-Tier Performance Among Peers
For broad knowledge and general reasoning tasks,as measured by benchmarks like MMLU,Claude Opus 4 performs at the very top tier,alongside models like GPT-4 and Gemini Ultra. Scores are often within a few percentage points,indicating that these leading models have all achieved a remarkable level of general intelligence. Opus 4 does show a particular edge on highly challenging reasoning benchmarks like GPQA Diamond and AIME,suggesting superior depth in certain types of complex logical and mathematical problem-solving.
For many common use cases involving question answering,summarization,and content generation across general topics,the qualitative differences between these top models might be subtle. The choice may then come down to specific nuances in output style,reliability on particular types of prompts,cost,latency,or the availability of specialized features like Claude 4's agentic toolkit.
Multimodality:A Current Gap for Claude 4
At its launch in May 2025,Claude 4 (Opus 4 and Sonnet 4) is primarily a text-based model. While it can process and generate text about images or other modalities if described in text,it lacks native,built-in capabilities for directly ingesting and generating image,audio,or video data.
This is an area where competitors like **OpenAI's GPT-4o and Google's Gemini family currently hold a distinct advantage**,having been designed as natively multimodal systems. These models can seamlessly understand and reason across text,images,audio,and (to varying degrees) video,enabling a wider range of applications,particularly in creative content generation,visual data analysis,and interactive user experiences that blend modalities.
Anthropic has indicated that multimodal capabilities are on their roadmap,and it's reasonable to expect future iterations of Claude to incorporate these. For now,users requiring strong native multimodal features might find competitor offerings more suitable,though Claude 4 can still be powerful if image/audio content is transcribed or described textually.
Safety and Alignment:Anthropic's Differentiating Focus
Anthropic has consistently emphasized AI safety and its **Constitutional AI** approach as a core differentiator. This involves training models not just on data but also on a set of explicit principles (the "constitution") to guide their behavior towards being helpful,harmless,and honest. The reported 65% reduction in shortcut behavior in Claude 4 is a tangible outcome of this focus.
While all major AI labs invest heavily in safety,Anthropic's explicit and research-driven methodology for alignment,including RLHF/RLAIF and its ASL (AI Safety Levels) framework,provides a distinct narrative. For organizations prioritizing auditable safety measures and demonstrable progress in reducing undesirable model behaviors,Claude 4's safety-centric design may be a compelling factor,especially as AI models become more autonomous and capable. The ASL-3 classification for Claude 4 also underscores Anthropic's transparency about the model's power and the associated responsibilities.
Strategic Positioning Summary:
Claude 4 strategically positions itself as the **undisputed leader for demanding software development tasks and the creation of sophisticated,autonomous AI agents**. Its unique combination of features like parallel tool use,persistent memory files,and validated long-duration performance,backed by SOTA benchmarks in coding and agentic tasks,carves out a compelling niche. While it competes fiercely with GPT-4 and Gemini in general reasoning (where it is also top-tier) and currently lags in native multimodality,its specialized strengths make it the go-to choice for organizations focused on developer productivity,complex automation,and reliable long-context processing where thoroughness and robust execution are paramount. Sonnet 4 further broadens this appeal by offering a high-value proposition for scaling these advanced capabilities more cost-effectively.
7. Transformative Industry Impact:Reshaping Workflows and Capabilities
The advanced capabilities embodied by Claude 4 are not just theoretical;they are poised to catalyze profound transformations across a multitude of industries. By moving beyond simple task automation to enabling complex,autonomous workflows and deep analytical insights,Claude 4 (Opus 4 and Sonnet 4) will likely redefine productivity,innovation,and even entire job roles.
7.1 Software Development & Engineering:A New Paradigm of AI Augmentation
Accelerating the Entire Development Lifecycle
The impact of Claude 4 on software development is expected to be revolutionary. Its SOTA performance on SWE-bench,ability to work autonomously for hours (Opus 4),and deep integration via Claude Code signal a shift where AI becomes an indispensable partner throughout the development lifecycle:
- Hyper-Efficient Coding & Prototyping: Generating high-quality code for complex features,algorithms,and entire modules from natural language specifications or high-level designs,drastically reducing initial development time.
- Autonomous Debugging & Root Cause Analysis: Analyzing vast codebases (200K context) and intricate error logs to pinpoint elusive bugs and propose accurate fixes,as demonstrated by its SWE-bench prowess. This includes understanding interactions in microservices or complex distributed systems.
- Advanced Automated Testing: Generating comprehensive unit tests,integration tests,and even end-to-end test scenarios,ensuring higher code quality and reliability. It can analyze code coverage and suggest areas needing more tests.
- Intelligent Code Refactoring & Modernization: Assisting with large-scale refactoring of legacy systems,migrating code to new languages or frameworks,optimizing performance bottlenecks,and improving code maintainability based on best practices.
- Proactive Code Review & Quality Assurance: Acting as an AI pair programmer within the IDE,Claude Code can offer real-time feedback on code style,potential bugs,security vulnerabilities,and adherence to coding standards,significantly offloading human reviewers.
- Comprehensive and Dynamic Documentation: Automatically generating and maintaining API documentation,code comments,architectural diagrams (described textually),and user guides that stay synchronized with code changes.
- Accelerated Onboarding and Knowledge Transfer: Helping new developers understand complex,unfamiliar codebases quickly by answering questions,explaining logic,and tracing dependencies.
This level of AI augmentation promises not just to speed up development but to elevate the quality and sophistication of software products,freeing human developers to focus on high-level architectural design,innovation,and solving the most challenging conceptual problems.

Projected Productivity Gains:
Early adopter reports and benchmark implications suggest potential for:
- 2-3x speedup in certain coding tasks.
- Up to **90% automated resolution** for specific bug classes.
- Significant reduction in time spent on manual code reviews.
- Faster developer onboarding onto complex projects.
7.2 Enterprise Automation:The Rise of True Digital Agents
Claude 4's powerful agentic features—Hybrid Reasoning,Parallel Tool Use,Enhanced Memory,and reduced shortcut behavior—are set to revolutionize enterprise automation. This moves beyond traditional Robotic Process Automation (RPA),which typically handles structured,repetitive tasks,into the realm of intelligent,adaptive "digital agents" capable of managing complex,dynamic,and multi-step business processes.

Key Enablers for Enterprise Agents:
Claude 4's architecture provides unique advantages:
- Parallel Tool Use: Efficiently interact with multiple enterprise systems (CRM,ERP,databases) concurrently.
- Enhanced Memory (Files): Maintain context and state across long-running processes (e.g.,month-end closing,complex customer cases).
- Hybrid Reasoning: Adapt strategies—quick responses for simple queries,deep analysis for complex decisions.
- Reduced Shortcuts: Ensure reliability and thoroughness in critical business workflows.
From RPA to Intelligent Process Automation (IPA):
Claude 4 enables a new tier of IPA,where agents can:
- Orchestrate End-to-End Business Processes: Autonomously manage complex sequences like order-to-cash,procure-to-pay,or customer onboarding. This could involve reading unstructured emails (using 200K context),extracting data,querying databases (via MCP connector),updating CRM/ERP systems,generating reports,sending notifications,and intelligently handling exceptions or escalations.
- Power Hyper-Personalized Customer Operations: Develop sophisticated virtual customer service agents that can understand nuanced queries,access extensive knowledge bases (memory files),interact with backend systems to retrieve customer-specific data (tools),provide human-quality resolutions,and learn from interactions to improve future service.
- Automate Complex Data Analysis & Reporting: Instruct an agent to gather data from disparate internal and external sources (parallel tool use),perform sophisticated analysis using its reasoning capabilities (or by invoking a code interpreter/analytics tool),identify key insights and trends,and compile comprehensive,presentation-ready reports.
- Transform Financial and Legal Operations: Automate tasks like advanced contract analysis and review (identifying risks,obligations,and non-standard clauses within large legal documents),complex compliance checks against evolving regulations,sophisticated fraud detection workflows involving multiple data points,and the generation of detailed financial statements or regulatory filings.
- Streamline Supply Chain Management: Monitor supply chains in real-time,predict disruptions by analyzing diverse data streams,automatically adjust inventory levels,and communicate with suppliers.
The ability of Claude 4 agents to operate autonomously for extended periods and reliably execute multi-step plans makes them suitable for taking over significant portions of knowledge work currently performed by humans,leading to substantial efficiency gains,cost reductions,and the potential to create entirely new service offerings.
7.3 Scientific Research & Discovery:Augmenting Human Ingenuity
For the scientific community,Claude 4's combination of advanced reasoning,a massive context window,sophisticated tool use,and persistent memory represents a powerful new instrument for accelerating the pace of discovery and innovation. It can act as a tireless research assistant,an insightful analytical partner,and a hypothesis generator.
Supercharging Knowledge Synthesis,Analysis,and Hypothesis Generation:
The sheer volume of scientific literature makes it impossible for human researchers to stay fully abreast of all developments,even within narrow sub-fields. Claude 4 can address this challenge:
- Automated Large-Scale Literature Reviews: Ingesting and synthesizing thousands of research papers (using its 200K context and potentially memory files for larger corpora) to identify key findings,track the evolution of concepts,pinpoint methodological flaws,highlight discrepancies in results,and summarize open questions or gaps in current knowledge.
- Novel Hypothesis Generation: By analyzing vast datasets of existing research,experimental results,and disparate information sources (via tools),Claude 4 may identify subtle patterns,correlations,or transdisciplinary connections that humans might overlook,leading to the formulation of novel,testable hypotheses.
- Experimental Design and Protocol Optimization: Assisting in the design of complex experimental protocols by reviewing prior art,suggesting alternative methodologies,identifying potential confounding variables,optimizing parameters (e.g.,for simulations run by a code interpreter tool),and even helping to draft ethics review board applications.
- Advanced Data Interpretation and Visualization: Helping researchers analyze complex experimental datasets (e.g.,genomic sequences,particle physics data,climate model outputs) by identifying significant patterns,performing statistical analyses (via code execution),and generating textual summaries or even code for visualizations of the findings.
- Cross-Disciplinary Knowledge Integration: Facilitating breakthroughs by connecting concepts,theories,and methodologies from seemingly unrelated scientific fields. For instance,applying principles from materials science to biological systems or insights from network theory to social sciences.
- Drafting Research Papers and Grant Proposals: Assisting in writing initial drafts of research papers,literature review sections,methodology descriptions,and even compelling narratives for grant proposals by organizing information,ensuring logical flow,and adhering to specific formatting guidelines.

Accelerating Discovery Cycles:
By automating many time-consuming aspects of knowledge work and data analysis,Claude 4 can free up researchers to focus on critical thinking,creative problem-solving,designing novel experiments,and interpreting results at a higher level. This has the potential to dramatically shorten discovery cycles in fields ranging from drug development and materials science to astrophysics and environmental science.
7.4 Impact Across Other Sectors
Beyond these core areas,Claude 4's influence will ripple through numerous other sectors:
- Content Creation & Marketing: Generating high-quality,long-form content,personalized marketing copy,scripts,and detailed analytical reports on campaign performance. Its strong reasoning can also help in developing complex content strategies.
- Education & Training: Creating personalized learning paths,sophisticated tutoring systems that can explain complex concepts in multiple ways,and tools for automated grading of nuanced assignments.
- Healthcare & Life Sciences: Assisting in analyzing medical literature,interpreting patient data (with appropriate privacy safeguards),accelerating drug discovery research,and potentially aiding in diagnostic processes.
- Finance & Investment: Performing complex financial modeling,analyzing market trends from diverse data sources,generating investment theses,and automating compliance reporting.
- Legal Tech: Enhancing tools for legal research,document review,contract analysis,and case summarization.
The common thread across these industries is Claude 4's ability to handle complexity,process large volumes of information,reason deeply,and operate with a greater degree of autonomy,thereby augmenting human expertise and automating sophisticated knowledge work.
8. Safety,Ethics & Responsible AI:Navigating the Power of Claude 4
As artificial intelligence models like Claude 4 achieve unprecedented levels of capability and autonomy,the imperative for robust safety measures,ethical considerations,and responsible development practices becomes paramount. Anthropic has consistently positioned AI safety at the core of its mission,pioneering approaches like **Constitutional AI**. With Claude 4,these efforts are more critical than ever,given the model's advanced reasoning,coding,and agentic potential.
8.1 Constitutional AI:The Bedrock of Claude's Behavior
Anthropic's flagship safety technique,Constitutional AI,plays a central role in shaping Claude 4's behavior. This approach involves:
- Defining a Constitution: A set of principles and rules derived from diverse sources such as universal human rights (e.g.,UN Declaration of Human Rights),terms of service of various platforms,and broad ethical guidelines. This constitution aims to make the AI helpful,harmless,and honest.
- AI-Supervised Fine-tuning (RLAIF): Instead of relying solely on extensive human feedback to align the model (RLHF),Anthropic uses AI models to critique and refine other AI models' responses based on the constitution. An AI model generates responses,another AI model critiques these responses against constitutional principles,and then the original model is fine-tuned based on these AI-generated critiques and preferences.
- Iterative Refinement: The constitution and the alignment process are continuously refined based on research,red-teaming (adversarial testing to find vulnerabilities),and feedback.
For Claude 4,this process has been further evolved,contributing to the reported 65% reduction in "shortcut behavior" and improved resistance to generating harmful or misleading content. The goal is to instill a more robust and generalizable sense of desirable behavior directly into the model.

8.2 Key Safety Features and Considerations for Claude 4:
- Improved Resistance to Harmful Content & Misuse: Claude 4 is trained to be more adept at detecting and refusing to generate harmful content (e.g.,hate speech,incitement to violence),instructions for illegal activities,or proliferable dangerous information (e.g.,related to bioweapons or cyberattacks).
- Enhanced Factuality and Reduced Hallucinations: While no LLM is perfectly immune to hallucination,efforts are continuously made to improve factuality,especially when combined with tool use for grounding answers in real-time data. The "reduced shortcut behavior" also contributes by encouraging more thorough,less speculative responses.
- Bias Mitigation: Ongoing work to identify and mitigate harmful societal biases that may be present in the vast training datasets. This involves careful data curation,algorithmic adjustments,and evaluation against fairness benchmarks. The aim is to promote more equitable and just outputs.
- Transparency and Explainability (Ongoing Research): While full explainability of such complex models remains a grand challenge,Anthropic is committed to research in this area. For users,this might manifest in clearer explanations of how a tool was used or a general line of reasoning,though deep mechanistic transparency is still evolving.
- Auditability and Monitoring: Deployments via platforms like Amazon Bedrock and Google Cloud Vertex AI,as well as Anthropic's API,typically provide logging and monitoring capabilities. This allows organizations to audit AI interactions for compliance,safety reviews,and to understand usage patterns.
8.3 ASL-3 Classification:Acknowledging Dual-Use Potential
Anthropic employs an internal AI Safety Levels (ASL) framework to classify its models based on their capabilities and potential risks. Claude 4 is reportedly classified as **ASL-3**. This classification signifies that the model possesses capabilities that could have significant societal impact and potential for misuse (dual-use potential),even if it's designed and trained for beneficial purposes.
An ASL-3 classification implies heightened safety protocols are necessary,both internally at Anthropic during development and testing,and externally for organizations deploying the model. This includes:
- Stricter access controls and monitoring for sensitive capabilities.
- Robust red-teaming to proactively identify potential misuse pathways.
- Clear guidance and terms of service for users regarding responsible deployment.
- Ongoing research into safeguards against catastrophic risks that could emerge from highly capable AI systems.
This classification reflects a mature and responsible approach,acknowledging that with great power comes great responsibility. It encourages a cautious and thoughtful approach to deploying such advanced AI.
8.4 The Shared Responsibility Model for Ethical AI
While Anthropic builds safety into Claude 4 at the foundational level,the ethical and responsible use of the AI ultimately involves a shared responsibility model:
- Anthropic's Role: Designing and training models with safety and ethics as core principles,providing transparency about capabilities and limitations,and continuously researching and implementing improved safety measures.
- Platform Providers' Role (e.g.,AWS,Google Cloud): Offering secure infrastructure,tools for monitoring and governance,and ensuring their platforms are used in accordance with responsible AI practices.
- Developers' and Deployers' Role:
- Implementing appropriate application-level guardrails and human oversight.
- Thoroughly testing AI applications for specific use cases,especially for fairness,bias,and accuracy.
- Being transparent with end-users about how AI is being used and the limitations of the AI.
- Considering the societal impact of their AI applications,including potential job displacement or the spread of misinformation.
- Adhering to legal and regulatory requirements related to AI and data privacy.
- End-Users' Role: Using AI tools responsibly,being aware of their potential for error,and critically evaluating AI-generated content.
The increasing power and autonomy of models like Claude 4 necessitate a multi-stakeholder approach to ensure that AI development and deployment are aligned with human values and societal benefit. Open discussion,ongoing research into AI ethics and alignment,and collaboration across industry,academia,and policymakers remain critical as we navigate this transformative technology.
Open Research Questions: Despite significant progress,AI safety and ethics remain active research areas. Key challenges include robustly preventing emergent harmful behaviors,ensuring fairness across diverse populations,achieving true model interpretability,and developing governance frameworks for superintelligent AI. Anthropic is actively engaged in research addressing these long-term challenges.
9. Future Outlook & Roadmap:The Continuing Evolution of Claude
The launch of Claude 4 is a major milestone,but it's clear that Anthropic views it as a significant step in an ongoing journey of AI development. The rapid iteration from Claude 3 to 3.5 and now to Claude 4 within a relatively short timeframe (roughly early 2024 for Claude 3,to May 2025 for Claude 4) signals an aggressive innovation cycle. Looking ahead,Anthropic's future roadmap is likely to focus on several key strategic thrusts,building upon Claude 4's strengths while addressing current limitations and pushing towards even more capable and safe AI.
9.1 Anthropic's Strategic Vision and Anticipated Development Priorities
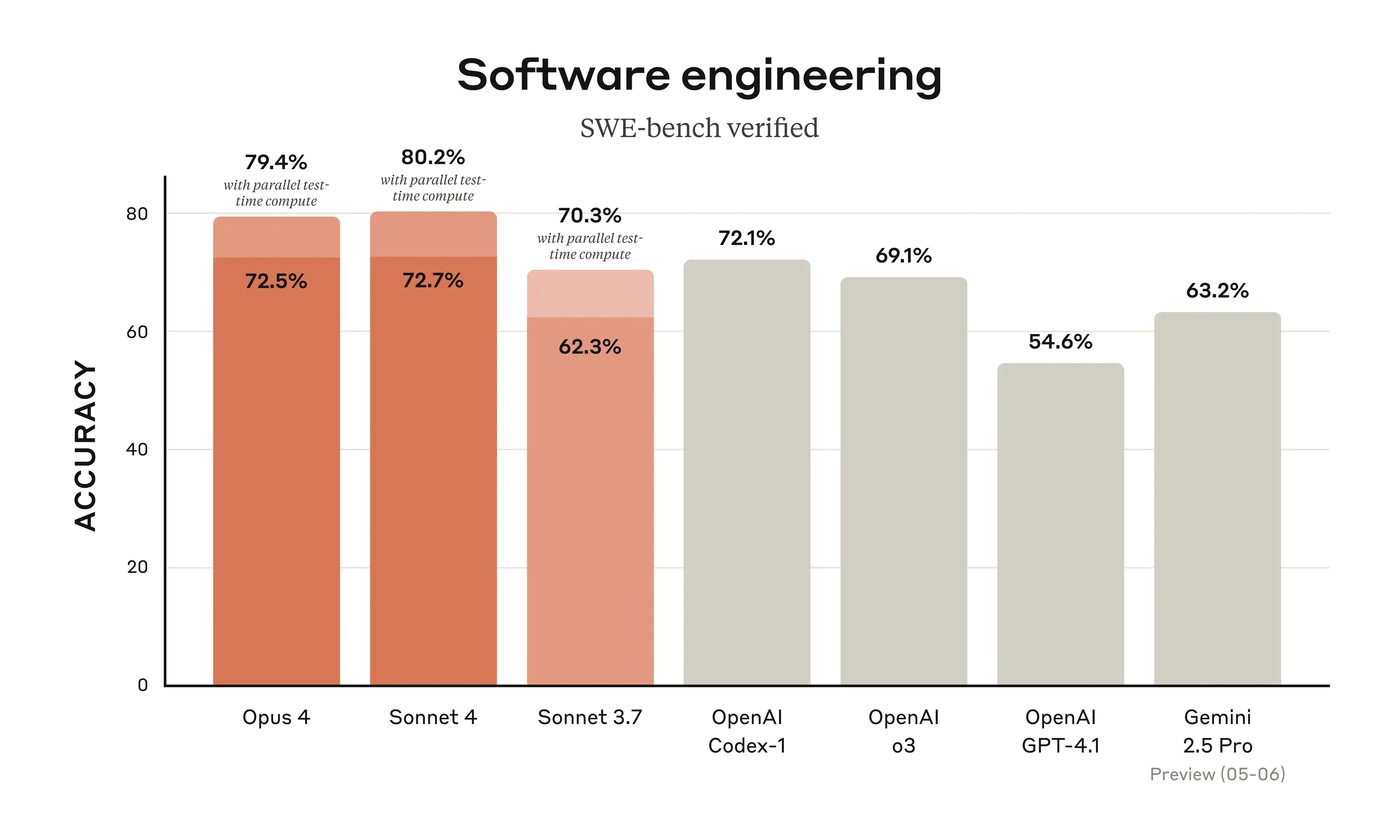
Key Areas for Future Development:
- Even More Powerful Agentic Autonomy: Further enhancing the ability of Claude agents to plan,execute complex multi-step tasks,learn from experience,and self-correct with even greater reliability and for longer durations. This could involve more sophisticated planning algorithms and more nuanced tool use.
- Refined Reasoning and World Models: Continuously improving logical deduction,abstract reasoning,common-sense understanding,and developing more robust internal "world models" to reduce errors,improve consistency,and handle ambiguity more effectively.
- Introduction of Native Multimodal Capabilities: A key area for future development will almost certainly be the integration of native vision,audio,and potentially other modalities. This would allow Claude to directly process and generate multimodal content,opening up a vast range of new applications and bringing it to parity with competitors in this domain.
- Enhanced Efficiency and Scalability (Cost Reduction): Ongoing efforts to make models more computationally efficient (both for training and inference) through techniques like architectural optimizations,improved quantization,and distillation. This would reduce operational costs and enable deployment on a wider range of hardware and in more resource-constrained environments.
- Further Advancements in Constitutional AI and Safety: As model capabilities increase,safety research must keep pace. This includes refining the constitution,improving alignment techniques,developing more robust defenses against misuse,and enhancing interpretability. The goal is to ensure that more powerful AI remains controllable and beneficial.
- Expansion of Developer Ecosystem and Enterprise Tools: Building out more comprehensive SDKs,APIs,fine-tuning capabilities,and integrations to make it easier for developers and enterprises to build sophisticated applications and custom agents on top of the Claude platform. This could include more specialized models for specific industries or tasks.
- Personalization and Customization: Offering more ways for users and organizations to customize Claude's behavior,knowledge,and style to better suit their specific needs,potentially through more advanced fine-tuning or few-shot adaptation techniques.
Anthropic's overarching goal appears to be the development of increasingly general and capable AI systems that can act as reliable,helpful,and harmless partners in complex human endeavors,all underpinned by a steadfast commitment to safety and responsible innovation.
9.2 Projected Development Timeline & Potential Milestones (Illustrative)
While Anthropic does not publish detailed long-term roadmaps,we can project an illustrative timeline based on past release cadences and industry trends. This timeline is speculative and subject to change based on research breakthroughs and strategic shifts.
Anticipated Near-Term and Medium-Term Milestones:
- Near-Term (Next 6-12 Months): Focus likely on refining Claude 4, expanding developer tools and enterprise-specific features (e.g., more robust MCP connectors, fine-tuning options for Sonnet 4), improving cost-performance for Sonnet 4, and gathering feedback to inform the next iteration. We might see incremental updates (e.g., "Claude 4.1") or specialized variants.
- Medium-Term (1-2 Years): Introduction of more significant architectural advancements, potentially including robust native multimodal capabilities (vision, audio). Further leaps in reasoning, planning, and agentic control. Continued scaling of context windows and memory capabilities, alongside ongoing safety research to manage the risks of even more powerful models. A "Claude 5" family could emerge in this timeframe.
- Long-Term (3+ Years): Anthropic's long-term ambitions likely extend towards more general forms of artificial intelligence (AGI or AGI-like systems), capable of human-level (or beyond) performance across a vast range of cognitive tasks. Addressing the profound safety and societal challenges associated with such advanced AI will be paramount.
9.3 Navigating the Competitive AI Frontier
Anthropic operates in a fiercely competitive environment. OpenAI, Google, Meta, and other research labs are all rapidly advancing their own models. This competitive pressure will continue to drive innovation at an accelerated pace. Key differentiating factors for Anthropic will likely remain:
- Its deep commitment to **AI safety research and responsible development**, particularly its Constitutional AI framework.
- Its focus on **enterprise-grade solutions** and robust agentic capabilities, as demonstrated by Claude 4.
- Its ability to attract top research talent and secure substantial funding for its ambitious R&D efforts (as evidenced by its strong revenue growth).
The future of the Claude series will depend on Anthropic's ability to continue innovating on these fronts, delivering measurable improvements in capability, safety, and usability, while adapting to the evolving needs of developers, enterprises, and society at large.
10. Conclusion: Claude 4 and the Dawn of Practical, Autonomous AI
The launch of Anthropic's Claude 4 model family on May 22, 2025, is far more than a routine product update; it signifies a pivotal moment in the trajectory of artificial intelligence. With the flagship **Claude Opus 4** and the versatile **Claude Sonnet 4**, Anthropic has delivered a suite of AI systems that don't just incrementally improve upon existing capabilities but fundamentally redefine what we can expect from AI in terms of practical application, sophisticated reasoning, and, most compellingly, **true autonomous operation**.
Throughout this comprehensive analysis, we've explored Claude 4's exceptional performance in demanding software engineering benchmarks like SWE-bench and its groundbreaking capabilities in autonomous terminal operations (Terminal-bench). These achievements, coupled with its advanced hybrid reasoning, parallel tool use, enhanced persistent memory via file access, and vast 200K+ token context window, position Claude 4 as a transformative force, particularly for developers and enterprises seeking to build the next generation of AI-powered solutions.
The strategic simultaneous launch on major cloud platforms like Amazon Bedrock and Google Cloud Vertex AI underscores Anthropic's commitment to making this power accessible, scalable, and integrable into the fabric of modern enterprise IT. This is AI designed not for a lab, but for the real world, ready to tackle complex challenges in software development, research, business process automation, and beyond.
However, the immense power of Claude 4 also brings to the forefront the profound responsibilities that accompany such advancements. Anthropic's unwavering focus on AI safety, epitomized by its Constitutional AI framework and ASL-3 classification for these models, is a critical and commendable aspect of this release. As AI systems become increasingly autonomous and capable of interacting with the world in complex ways, the guardrails of safety, ethics, and responsible deployment are not just important—they are indispensable.
Claude 4 challenges us to reimagine workflows, to rethink the boundaries of automation, and to consider new paradigms of human-AI collaboration. It offers a glimpse into a future where AI agents can reliably undertake complex, multi-hour tasks, assist in profound scientific discovery, and drive unprecedented levels of productivity. The age of truly practical, autonomous AI is no longer a distant theoretical concept; with models like Claude 4, it is actively unfolding before us, bringing with it both immense opportunity and a solemn duty to navigate its development with wisdom and foresight.
Agentic Revolution
Unprecedented autonomous work duration and parallel tool use for complex task execution.
SOTA Performance
New industry records in coding (SWE-bench) and advanced reasoning (GPQA Diamond) benchmarks.
Safety-Driven Design
Built on Constitutional AI principles with ASL-3 classification, emphasizing responsible AI.
11. Frequently Asked Questions (FAQ) about Claude 4
What are the main differences between Claude Opus 4 and Claude Sonnet 4? +
Claude Opus 4 is Anthropic's flagship model, designed for maximum performance on the most complex tasks. It offers the highest levels of reasoning, coding proficiency (especially in "High Compute" mode), and agentic capabilities. It's ideal for research, cutting-edge software development, and strategic analysis where peak intelligence is required. Claude Sonnet 4 is engineered for a balance of high performance and cost-effectiveness. It's very capable (e.g., SOTA on SWE-bench for its class) but optimized for speed and efficiency, making it suitable for large-scale enterprise deployments, high-volume coding tasks, and intelligent automation where throughput and value are key. Both share core features like the 200K context window and parallel tool use.
How does Claude 4's "Enhanced Memory" with file access work? +
When granted permission (via the Files API), Claude 4 can create and interact with "memory files" on a local or specified file system. This allows it to store key facts, summaries of previous interactions, project details, or intermediate results from long tasks. This information persists across sessions, enabling the model to "remember" context for ongoing projects or very long analyses that exceed even its 200K token in-context memory. The model intelligently decides what to write to these files and when to retrieve information from them to inform its current operations, acting as a form of persistent, structured long-term memory.
What is "Parallel Tool Use" and why is it significant? +
Parallel tool use allows Claude 4 to invoke and manage multiple external tools (like API calls, code execution, web searches, or database queries via the MCP Connector) simultaneously, rather than one after another. This is significant because it can drastically speed up complex tasks that require information or actions from multiple sources. For example, an AI agent could concurrently fetch data from three different APIs instead of doing it sequentially. This enhances efficiency, enables more complex agentic behaviors, and allows for faster real-time decision-making by gathering diverse information in parallel.
How does Claude 4 compare to models like GPT-4o or Gemini 1.5 Pro? +
Claude 4 (especially Opus 4) establishes leadership in specific areas like complex coding (SWE-bench) and autonomous agentic tasks (Terminal-bench, 7+ hour work duration). Its general reasoning (MMLU, GPQA) is top-tier, competitive with GPT-4 series and Gemini Ultra. However, GPT-4o and Gemini 1.5 Pro currently lead in native multimodal capabilities (processing images, audio directly). Gemini 1.5 Pro also offers a larger raw context window (1M tokens vs Claude 4's 200K). Claude 4's strengths lie in its specialized agentic architecture, persistent memory files, and focus on reducing "shortcut" behaviors, making it highly reliable for complex, long-duration tasks. The best choice depends on the specific priorities of the use case.
What does an "ASL-3" classification mean for Claude 4? +
ASL (AI Safety Level) is Anthropic's internal framework for categorizing AI models based on their capabilities and potential risks. An ASL-3 classification for Claude 4 indicates that it is a highly capable model with significant potential for beneficial applications, but also possesses capabilities that could be misused if not handled responsibly (dual-use potential). This triggers stricter safety protocols during development, thorough red-teaming, and necessitates responsible deployment practices by users to mitigate potential harms. It reflects Anthropic's commitment to transparency about model power and safety.
Where can I access Claude 4 models? +
Claude Opus 4 and Claude Sonnet 4 are available through the official Anthropic API. Additionally, they were launched simultaneously on major cloud platforms, including Amazon Bedrock and Google Cloud Vertex AI. This multi-platform availability is designed to make it easier for developers and enterprises to integrate Claude 4 into their existing workflows and infrastructure. Specific availability and pricing details can be found on Anthropic's website and the respective cloud provider platforms.
Experience the Future of AI with Claude 4
Ready to harness the state-of-the-art capabilities of Claude Opus 4 and Sonnet 4 for your most demanding coding, reasoning, and autonomous agent tasks? Explore the documentation, dive into the APIs, and start building transformative AI solutions today.
For the latest updates, research papers, and safety information, please visit the official Anthropic website.